Word net的問題,我們搜遍了碩博士論文和台灣出版的書籍,推薦Hains, Todd R.寫的 Martin Luther and the Rule of Faith: Reading God’s Word for God’s People 和的 Ben the Sea Lion都 可以從中找到所需的評價。
另外網站WordNet and the Organization of Lexical Memory - SpringerLink也說明:WordNet, an on-line lexical database for English based on ... It is suggested that semantic networks like Word-Net would be valuable aids in teaching second ...
這兩本書分別來自 和所出版 。
國立陽明交通大學 材料科學與工程學系所 鄒年棣所指導 許家維的 基於深度學習進行電池性質預測 (2021),提出Word net關鍵因素是什麼,來自於鋰離子電池、老化因子、剩餘壽命、深度學習、特徵篩選、時序資料處理。
而第二篇論文國立中正大學 資訊管理系研究所 胡雅涵、李珮如所指導 宋昇峯的 以監督式機器學習探討電子病歷中非結構化資料對早期預測中風後功能復原後果之價值 (2021),提出因為有 急性缺血性中風、電子病歷、功能復原後果、機器學習、敘述式臨床紀錄、自然語言處理、風險模型、預測的重點而找出了 Word net的解答。
最後網站ConvertPDFtoWord.net: Convert your PDF file into Word則補充:Convert your PDF into Word document for free.
Martin Luther and the Rule of Faith: Reading God’s Word for God’s People
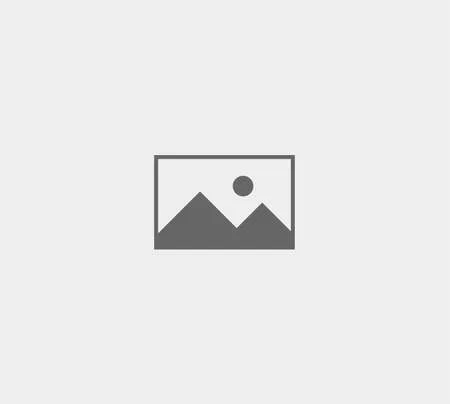
為了解決Word net 的問題,作者Hains, Todd R. 這樣論述:
Martin Luther is a giant among the church’s theologians. He is especially known for advocating views such as justification by faith and the priesthood of all believers, which challenged the late-medieval Roman Catholic Church.Yet the reading of God’s Word was what Luther considered his primary task
as a theologian--and as a Christian. Though he is often portrayed as reading the Bible with a bare approach of sola Scriptura, without any reference to or concern for previous generations’ interpretation, the truth is much more complicated.In this volume in IVP Academic’s New Explorations in Theolog
y (NET) series, Reformation scholar Todd R. Hains considers how Luther read the Bible according to the rule of faith, which guided his interpretation of the text by the church’s established practice of hermeneutics as reflected in the Apostles’ Creed and the church’s catechism.This study will helpfu
lly complicate your view of Luther and bring clarity to your own reading of God’s Word.
Word net進入發燒排行的影片
"Everything You Do" is out now ⬇︎
各配信サイトはこちら
https://linkco.re/Q2U5mrTr?
Last year November, my shooting crew and I took a trip to the beautiful alps of Japan and created an aesthetic video of the views. I love the mountains. A trip back to nature always makes me feel grateful, content, and gives me a sense of nostalgia. My hopes were to recreate such emotion in the song and video.
I hope you enjoy!
Music: Sincere Tanya and Kiyomaro
Lyrics: Sincere Tanya and Kiyomaro
Produce: Kiyomaro
シンシア ターニャ / Sincere Tanya
Instagram: https://www.instagram.com/sinceretanyaa/
Twitter: https://www.twitter.com/sincere_tanya
Kiyomaro
Instagram: https://www.instagram.com/kiyomaro556/
------------------------------------
[Videography]
Directer Of Photography:Wataru Sato
twitter: https://www.twitter.com/locowataru310
HP: https://www.locowataru5.net
Still Photography: Kazuyuki Nagata
instagram: https://www.instagram.com/wacoh_
HP:https://wacoh-jp.com/
Camera Assistant: Asami Nishizu
twitter: https://www.twitter.com/acchan0831
------------------------------------
[Lyrics]
Just what I can see
Just what you can be
Whispers what you lost
Memories leaking
Touch all you cannot see
Hide all that you can be
Remembering the cost
We can reach what we please
Every morning I'm
Imagining what's happening
Looking at the sky
I'd whisper a "hello"
Counting all times I've cried
Counting seconds of it all
I'm thinking all the time
How blessed I am being with you
Counting all times I've laughed
Counting seconds of it all
Everyday just keeps on going
But I know I will be walking it with you
The road that we walk on
Every step I take with you
I know how to hold on
And I hope you feel it too
Every word that you're speaking
Every sound your movement makes
Your everything's uplifting
And you're leading me the way
次の朝
目覚めた君にも
風にのる
この歌届くかな
あふれそうなくらい
集めた日々が
色めいてく
秋に想う木々のように
Times like this
I'm swimming in my memories
I think a bit
How I love you next to me
Times like this
I'm so glad to be with you
This happiness
I'm so glad to share with you
君と歩く足音のリズム
愛の音になって
やまびこと重なる
君の言葉
一つ一つが
歌声に変わって
空は染まってゆく
基於深度學習進行電池性質預測
為了解決Word net 的問題,作者許家維 這樣論述:
鋰離子電池作為常見的儲能設備,廣泛應用於終端設備上且藉由電池管理系統進行監控確保電池老化程度仍可應付工作所需。然而電池在使用初期並無明顯老化特性的反應,因此對於使用過的電池無法很好評估預期壽命以至於材料的浪費或設備的異常(Early failure)。本研究利用時序資料連續性進行資料擴增更同時對神經網路潛空間進行正則化,並透過包含篩選器與預測器的神經網路架構在僅有少量循環的量測數據下,預測電池產品壽命、剩餘使用壽命、充電所需時間、放電時的電壓電量變化曲線等。其中,僅測量一個充放電完整循環的數據,就能提供僅有57週期方均根誤差的產品壽命預測。本研究亦同時引入注意力機制於此框架中達成僅使用若干個
循環的測量資料便可預測整個電池的產品週期放電電量、放電功耗等特性。
Ben the Sea Lion

為了解決Word net 的問題,作者 這樣論述:
Tsimshian storyteller and artist Roy Henry Vickers shares an adventure from his childhood in the Indigenous Pacific Northwest village of Kitkatla.When Uncle Johnny accidentally catches an orphaned sea lion pup in his fishing net, young Roy and his cousin Bussy take responsibility for nursing the
tiny creature back to health. They name the pup Ben, short for Teeben--the Tsimshian word for sea lion. With the boys’ loving care, Ben eats and eats and grows and grows, getting up to all sorts of fun in Kitkatla, including towing the boys in their skiff and showing local dogs who is boss! Eventual
ly, Ben must return to the wild, leaving his human friends to remember him fondly.Fifteen original illustrations by Roy Henry Vickers accompany the text, capturing the beauty of the West Coast and the richness of village life. Ben the Sea Lion will delight readers of all ages.
以監督式機器學習探討電子病歷中非結構化資料對早期預測中風後功能復原後果之價值
為了解決Word net 的問題,作者宋昇峯 這樣論述:
中風是導致成人殘障的重要原因,中風功能復原後果的精準預測,能協助病人及家屬及早準備後續照顧事宜,衛生政策制定者也能依此預測結果適切規劃人力與資源,以投入中風病人的急性後期與中長期照護。目前的中風功能復原後果預測模型皆是以結構化資料建立,甚至最新使用數據驅動方式發展的機器學習預測模型依然是以結構化資料為主。相對的,照顧病人所製作的大量敘述式病歷文字紀錄,即非結構化資料,反而甚少被使用。因此,本研究的目的,即是使用監督式機器學習來探討非結構化臨床文字紀錄於急性缺血性中風後之初期預測功能復原後果之應用價值。在6176位2007年10月至2019年12月間因急性缺血性中風住院之病人中,共3847位病
人符合本研究之收案/排除條件。我們使用自然語言處理,萃取出住院初期之醫師紀錄及放射報告中之臨床文字紀錄,並且實驗了不同文字模型與機器學習演算法之組合,來建構中風功能復原後果的預測模型。實驗發現使用醫師紀錄時,操作特徵曲線下面積為0.782至0.805,而使用放射報告時,曲線下面積為0.718至0.730。使用醫師紀錄時,最好的組合為詞頻-倒文件頻加上羅吉斯迴歸,而使用放射報告時,最好之組合為基于轉換器的雙向編碼器表示技術加上支持向量機。這些基於純文字的機器學習預測模型並無法勝過傳統的風險模型,這些傳統模型的曲線下面積為0.811至0.841。然而,不管是以曲線下面積、重分類淨改善指標、或整合式
區辨改善指標來評估,臨床文字紀錄中的資訊的確可以增強傳統風險模型的預測效能。本研究之結論為,電子病歷中的非結構化文字經過自然語言處理後,不僅可以成為另類預測中風功能復原後果的工具,更可以增強傳統風險模型的預測效能。透過演算法來自動擷取並整合分析結構化與非結構化資料,將能提供醫師更好的決策支援。
想知道Word net更多一定要看下面主題
Word net的網路口碑排行榜
-
#1.Word .NET库组件(28):设置Word 表格的格式 - 慧都
本系列教程将为大家带来Spire.Doc for .NET在使用过程中的各类实际操作,本篇文章介绍了如何设置Word 表格的格式。 於 www.evget.com -
#2.(PDF) WordNet-Affect: an Affective Extension of WordNet
In this paper we present a linguistic resource for a lexical representation of affective knowledge. This re- source (named WORDNET-AFFECT) was ... 於 www.researchgate.net -
#3.WordNet and the Organization of Lexical Memory - SpringerLink
WordNet, an on-line lexical database for English based on ... It is suggested that semantic networks like Word-Net would be valuable aids in teaching second ... 於 link.springer.com -
#4.ConvertPDFtoWord.net: Convert your PDF file into Word
Convert your PDF into Word document for free. 於 www.convertpdftoword.net -
#5.Open English WordNet – 2021 Edition Released - ELEXIS
Incorporation of sentence template information; Adoption of Global WordNet Association v1.1 format; Reorganization of syntactic behaviors to reduce file sizes ... 於 elex.is -
#6.WordNet: a lexical database for English - ACM Digital Library
WordNet 1 provides a more effective combination of traditional lexicographic information and modern computing. WordNet is an online lexical ... 於 dl.acm.org -
#7.【Python&NLP】关于WordNet,我的一些用法和思路(二)
上次写了一些关于Python中nltk库里WordNet文件的具体内容,这次介绍一下笔者对中英文WordNet的理解,以及一些初步的应用。 贰、我对WordNet的理解和 ... 於 zhuanlan.zhihu.com -
#8.wordnet | TensorFlow Datasets
WordNet is a large lexical database of English. Nouns, verbs, adjectives and adverbs are grouped into sets of cognitive synonyms (synsets), each expressing ... 於 www.tensorflow.org -
#9.WordNet - Devopedia
WordNet is a network of words linked by lexical and semantic relations. Nouns, verbs, adjectives and adverbs are grouped into sets of cognitive synonyms, called ... 於 devopedia.org -
#10.English Thesaurus (WordNet) on the App Store
... English Thesaurus (WordNet). Download English Thesaurus (WordNet) and enjoy it on your iPhone, iPad, iPod touch, or Mac OS X 10.15 or later. 於 apps.apple.com -
#11.Word Net - C2 wiki
WordNet is a very interesting "model" of (the English) language out of Princeton that links word definitions and synonyms together in a navigable network ... 於 wiki.c2.com -
#12.Wordnet - Definition, Meaning & Synonyms - Vocabulary.com
any of the machine-readable lexical databases modeled after the Princeton WordNet. 於 www.vocabulary.com -
#13.Chinese Wordnet - 中文詞彙網路沿革 - LOPE Lab
中文詞彙網路(Chinese Wordnet, 以下簡稱中文詞網) 計畫,目的是在提供完整的中文詞義(sense) 區分與詞彙語意關係知識庫。我們相信詞義的區分與表達,必須建立在完善的 ... 於 lope.linguistics.ntu.edu.tw -
#14.word net - Wikidata
wordnet. In more languages. Spanish. No label defined. No description defined. Traditional Chinese. No label defined. No description defined. 於 www.wikidata.org -
#15.Net Definition & Meaning - Dictionary.com
Net definition, a bag or other contrivance of strong thread or cord ... for catching fish, birds, or other animals: a butterfly net. ... Save This Word! 於 www.dictionary.com -
#16.Serbian Wordnet - META-SHARE
Serbian WordNet (SrpWN) represents a lexical semantic network, containing synsets with glosses and various semantic relations, such as antonymy, meronymy, ... 於 metashare.elda.org -
#17.WordNet介绍,一个开放知识图谱_Norstc的博客
同义词集合(synonymy set)可以看作是词形(word form)之间一种具有中心角色的语义关系。WordNet的2.0版本中,有115424个同义词集合,其中名词同义词 ... 於 blog.csdn.net -
#18.The Word Network
The largest African-American Religious Network in the world. bannermobile. Broadcast ... The Word Network LiveStream . Video Player is loading. 於 www.thewordnetwork.org -
#19.英文詞彙知識庫WordNet如何應用於英文學習- 以glycogen(肝糖 ...
WordNet 目前的版本為Windows WordNet 2.1及Unix WordNet 3.0(網址:http://wordnet.princeton.edu/wordnet/download/current-version/ )。它包含語義訊息(Semantic ... 於 epaper.naer.edu.tw -
#20.Wordnet與字義關聯 - iT 邦幫忙
NLTK中包括英語WordNet,共有155,287個詞和117,659個同義詞集合。 我們將以尋找同義詞和它們在WordNet中如何訪問開始。 from nltk.corpus import wordnet as wn. 意義 ... 於 ithelp.ithome.com.tw -
#21.WordNet: An Electronic Lexical Database | MIT Press eBooks
with a preface by George Miller WordNet, an electronic lexical database, is considered to be the most important resource available to researchers in ... 於 ieeexplore.ieee.org -
#22.[PDF] Thai WordNet Construction - Semantic Scholar
This paper describes semi-automatic construction of Thai WordNet and the applied method for Asian wordNet. Based on the Princeton WordNet, ... 於 www.semanticscholar.org -
#23.93. WordNet: Principles, developments and applications
Wordnet is a lexical semantic database that implements a relational model of meaning for words. Concepts are formed by sets of. 於 www.degruyter.com -
#24.Wordnet | Fibra Óptica com qualidade!
VANTAGENS DE SER Wordnet. Veja nossas principais vantagens e venha você também para a Wordnet. Sem custo de Telefone. Wi-FI Premium GRÁTIS. Fibra Óptica ... 於 www.wordnet.com.br -
#25.WordNet TV – Sharing God's Love Through Media
Links · Donate Now · Offer Wednesday Mass · Fr. Padovani's Books and CDs · Newsletter · Current Program Guide · FAQ · Links / Media Partners · Wordnet Studio ... 於 wordnet.tv -
#26.(PDF) Paperon Word Net - Academia.edu
Dravidian WordNet will be a multilingual lexical database with wordnets for four major Dravidian languages, which will be structured along the same lines as the ... 於 www.academia.edu -
#27.Net Price Calculator | Financial Assistance
To use the Net Price Calculator, please enable Javascript. Back to Top. University of the Incarnate Word Footer Logo. 於 www.uiw.edu -
#28.NLP | Synsets for a word in WordNet - GeeksforGeeks
WordNet is the lexical database i.e. dictionary for the English language, specifically designed for natural language processing. 於 www.geeksforgeeks.org -
#29.WordNet::QueryData - direct perl interface to WordNet database
It requires the WordNet package (http://www.cogsci.princeton.edu/~wn/). It allows the user direct access to the full WordNet semantic lexicon. All parts of ... 於 metacpan.org -
#30.Sanskrit WordNet
About. The Sanskrit WordNet is an on-going collaboration between the University of Pavia and the University of Exeter, under the joint direction of William ... 於 sanskritwordnet.unipv.it -
#31.Wordnet with NLTK - Python Programming Tutorials
WordNet is a lexical database for the English language, which was created by Princeton, and is part of the NLTK corpus. You can use WordNet alongside the ... 於 pythonprogramming.net -
#32.WordNet - Wiktionary
EtymologyEdit · word + net. Proper nounEdit. WordNet. (linguistics, artificial intelligence) A particular wordnet, a semantically structured lexical ... 於 en.wiktionary.org -
#33.WordNet
WordNet ® is a large lexical database of English. Nouns, verbs, adjectives and adverbs are grouped into sets of cognitive synonyms (synsets), each expressing a ... 於 wordnet.princeton.edu -
#34.The first two levels of the WordNet 1.5 ontology of noun ...
WordNet is a public domain on-line lexical reference system developed at Princeton University. In version 1.5, some 120,000 word forms of English nouns, ... 於 www-sop.inria.fr -
#35.Introduction to WordNet: An On-line Lexical Database
Abstract. WordNet is an on-line lexical reference system whose design is inspired by current psycholinguistic theories of human lexical ... 於 academic.oup.com -
#36.Software: Open English WordNet - 2021 Edition Released
LINGUIST List 32.3626. Tue Nov 16 2021. Software: Open English WordNet - 2021 Edition Released. Editor for this issue: Everett Green ... 於 linguistlist.org -
#37.WordNet - 维基百科,自由的百科全书
WordNet 是一个由普林斯顿大学认识科学实验室在心理学教授乔治·A·米勒的指导下建立和维护的英语字典。开发工作从1985年开始,从此以后该项目接受了超过300万美元的 ... 於 zh.wikipedia.org -
#38.Home - WordNet - Tune in for All Good News. All The Time.
WordNet is a radio and television station delivering the life-changing Gospel of Jesus to Charlotte, Salisbury, Gastonia and beyond. 於 wordnet.org -
#39.中文詞彙網路CWN 2.0
中文詞彙網路(Chinse Wordnet,CWN),是一項試圖解決詞義(sense)以及詞彙語意關係(lexical semantic relations)的語言知識資源。中文詞網的核心元素是中文詞彙的 ... 於 lopentu.github.io -
#40.Overview of WordNet - YouTube
WordNet - Natural Language Processing With Python and NLTK p.10 · Word Sense Disambiguation · Social network analysis - Introduction to structural ... 於 www.youtube.com -
#41.C# .NET Word Document Processing API - Aspose
C# ASP.NET VB.NET library to work with Word files. Create edit convert DOC, DOCX, PDF, RTF, ODT, TXT, MOBI, HTML, JPG, PNG and many more formats. 於 products.aspose.com -
#42.WordNet - 中文百科知識
WordNet 是由Princeton 大學的心理學家,語言學家和計算機工程師聯合設計的一種基於認知語言學的英語詞典。它不是光把單詞以字母順序排列,而且按照單詞的意義組成 ... 於 www.easyatm.com.tw -
#43.wordnet - npm
English-English dictionary with 140k+ words from WordNet.. Latest version: 2.0.0, last published: 10 months ago. Start using wordnet in your ... 於 www.npmjs.com -
#44.The Word "Net" in Example Sentences - Page 1
This page requires JavaScript. · English Sentences Focusing on Words and Their Word Families The Word "Net" in Example Sentences Page 1. 於 www.manythings.org -
#45.The Open English WordNet - GitHub
Open English WordNet is a lexical network of the English language grouping words into synsets and linking them according to relationships such as hypernymy, ... 於 github.com -
#46.Ontology Learning Using Word Net Lexical Expansion and ...
WordNet is an online semantic dictionary, partitioning the lexicon into nouns, verbs, adjectives, and adverbs [23]. Some current researchers extract words from ... 於 www.intechopen.com -
#47.dolthub/word-net
WordNet is a lexcial database of Synonym Sets (or Synsets). Synsets are related to each other through lexical pointers. 於 www.dolthub.com -
#48.wordnet - PyPI
`Clone this repo` and install wordnet module using this script, $python setup.py install 1. To create a `TF-IDF` structure file for every ... 於 pypi.org -
#49.WordNet, EuroWordNet and Global WordNet | Cairn.info
The database, called WordNet, was organized around the notion of a synset between which semantic relations are expressed. A synset is a set of words with the ... 於 www.cairn.info -
#50.Wordwall | Create better lessons quicker
The easy way to create your own teaching resources. Make custom activities for your classroom. Quizzes, match ups, word games, and much more. 於 wordwall.net -
#51.wordnet | RubyGems.org | your community gem host
wordnet 1.1.1. This library is a Ruby interface to WordNet®[http://wordnet.princeton.edu/]. WordNet® is an online lexical reference system whose design is ... 於 rubygems.org -
#52.WordNet: A Lexical Database for English - ACL Anthology
WordNet : A Lexical Database for English. In Human Language Technology: Proceedings of a Workshop held at Plainsboro, New Jersey, March 8-11, 1994. 於 aclanthology.org -
#53.Wordnet Bahasa
Wordnet Bahasa ... We are creating a large scale, freely available, semantic dictionary of the Malay languages (currently Malaysian and Indonesian). This is the ... 於 wn-msa.sourceforge.net -
#54.Building a Wordnet for Turkish - CORE
All six wordnets are linked to Princeton. WordNet, to wordnets built under the EuroWordNet project [3] and to any other wordnet linked to the Interlingual Index ... 於 core.ac.uk -
#55.Word Net - SlideShare
Structured lexicons and Lexical semantics Especially WordNet ® See D Jurafsky & JH Martin: Speech and Language Processing , Upper Saddle River NJ (2000): ... 於 www.slideshare.net -
#56.WordNet: An Electronic Lexical Database - 第 23 頁 - Google 圖書結果
Chapter 1 Nouns in WordNet George A. Miller WordNet ( version 1.5 ) contains almost 80,000 noun word forms organized into some 60,000 lexicalized concepts . 於 books.google.com.tw -
#57.wordnet - Rust - Docs.rs
API documentation for the Rust `wordnet` crate. ... Senses are different definitions or etymologies for a word. SenseRef. refers to the actual text of a ... 於 docs.rs -
#58.手把手教你NLTK WordNet使用方法 - 博客园
最近看了WordNet,0基础入门,简单记下笔记。因为本身不是学自然语言处理的,好多名词不是特别清楚,现有的博客读的晕乎乎,所以重新写了这个, ... 於 www.cnblogs.com -
#59.Net Definition & Meaning - Merriam-Webster
Word History. Etymology. Noun (1) and Verb (1). Middle English nett, from Old English; akin to Old High German nezzi net. Adjective. 於 www.merriam-webster.com -
#60.WordNet - Kaggle
<package id="wordnet" name="WordNet" version="3.0" license="Permission to use, copy, modify and distribute this software and database and its documentation ... 於 www.kaggle.com -
#61.English WordNet: A new open-source wordnet for English
Wordnets are a form of dictionary that aim to make information more easily processable for computers. The primary unit of a wordnet is a set of synonyms or a ... 於 lexicala.com -
#62.हिन्दी शब्दतंत्र (Hindi Wordnet) - CFILT - IITB
The Hindi WordNet is a system for bringing together different lexical and semantic relations between the Hindi words. It organizes the lexical information ... 於 www.cfilt.iitb.ac.in -
#63.Word Net - TOEFL Dancisuji : Qiu contingent Gong Liying 118 ...
Word Net - TOEFL Dancisuji : Qiu contingent Gong Liying 118(Chinese Edition) [李秋然,龚丽英] on Amazon.com. *FREE* shipping on qualifying offers. 於 www.amazon.com -
#64.47 Synonyms & Antonyms for NET - Thesaurus.com
Find 47 ways to say NET, along with antonyms, related words, and example sentences at Thesaurus.com, the world's most trusted free thesaurus. 於 www.thesaurus.com -
#65.TheWord.net
沒有這個頁面的資訊。 於 www.theword.net -
#66.如何自動化Word 以從Visual Basic .NET 執行合併列印
本文示範如何使用Microsoft Office Word,從Microsoft Visual Basic .NET 使用自動化來建立合併列印檔。 其他相關資訊. 自動化是一種程式,可讓以Visual ... 於 learn.microsoft.com -
#67.Language modelling makes sense: Propagating
... through WordNet for Full-Coverage Word Sense Disambiguation ... Our approach focuses on creating sense-level embeddings with full-coverage of WordNet, ... 於 www.aclweb.org -
#68.OfficeIMO - Microsoft Word .NET Library - Evotec
What it's all about. This is a small project (under development) that allows to create Microsoft Word documents (.docx) using .NET. 於 evotec.xyz -
#69.Wordnet_百度百科
WordNet 是由Princeton 大学的心理学家,语言学家和计算机工程师联合设计的一种基于认知语言学的英语词典。它不是光把单词以字母顺序排列,而且按照单词的意义组成 ... 於 baike.baidu.com -
#70.Wordnet - Free Dictionary Org - Apps on Google Play
Wordnet 3.0 English Dictionary for offline use in Free Dictionary Org application. WordNet is a lexical database for the English language. 於 play.google.com -
#71.Semi-automatic WordNet Linking using Word Embeddings
Thus techniques that can aid the experts are desirable. In this paper, we propose an approach to link wordnets. Given a synset of the source ... 於 arxiv.org -
#72.A Complete Guide to Using WordNET in NLP Applications
WordNET is a lexical database of words in more than 200 languages in which we have adjectives, adverbs, nouns, and verbs grouped differently ... 於 analyticsindiamag.com -
#73.Word Senses and WordNet - Stanford University
word sense a discrete representation of one aspect of the meaning of a word. In this chapter we discuss word senses in more detail and introduce WordNet, ... 於 web.stanford.edu -
#74.The Structure of a Wordnet - wn 0.9.2 documentation
A wordnet is an online lexicon which is organized by concepts. The basic unit of a wordnet is the synonym set (synset), a group of words that all refer to the ... 於 wn.readthedocs.io -
#75.wordnet 3.0 — Packages - GNU Guix
WordNet is a large lexical database of English. Nouns, verbs, adjectives and adverbs are grouped into sets of cognitive synonyms (synsets), each expressing ... 於 guix.gnu.org -
#76.WordNet: A Lexical Taxonomy of English Words
A really useful lexical resource is WordNet. Its unique semantic network helps us find word relations, synonyms, grammars, etc. 於 towardsdatascience.com -
#77.wordnet - Homebrew Formulae
wordnet. Install command: brew install wordnet. Lexical database for the English language. https://wordnet.princeton.edu/. License: Cannot Represent. 於 formulae.brew.sh -
#78.English WordNet 2019 – An Open-Source WordNet for English
WordNet (Miller, 1995; Fellbaum, 1998) is one of the most widely-used language resources in natu- ral language processing and continues to find us- age in a ... 於 john.mccr.ae -
#79.Estonian Wordnet - Arvutilingvistika
Estonian Wordnet can be browsed in Teksaurus or WordTies. ... The atom of a wordnet-type thesaurus is a synonym set (also called a synset), ... 於 www.cl.ut.ee -
#80.WordNet - MIT Press
WordNet is an on-line lexical reference system whose design isinspired by current psycholinguistic theories of human lexical memory;version 1.6 is the most ... 於 mitpress.mit.edu -
#81.WN-Affect - WordNet Domains
WordNet -Affect is an extension of WordNet Domains, including a subset of synsets suitable to represent affective concepts correlated with affective words. 於 wndomains.fbk.eu -
#82.Word .NET - Word API - Independentsoft
NET is Microsoft Word compatible API for .NET Framework / .NET Core. The API allows you to easy create, read, modify and parse Word documents without to use ... 於 independentsoft.de -
#83.Latvian WordNet
The word senses are linked into 5331 synonym sets, and there are 3560 relations between them. The synonym sets are also linked to the English Princeton WordNet. 於 wordnet.ailab.lv -
#84.NLTK 初學指南(三):基於WordNet 的語義關係表示法 - Medium
WordNet 是一個以英文為主的語義網絡,在普林斯頓大學認知科學實驗室的心理學教授 George A. Miller 的指導下建立和維護的是一個由「字詞— 語義」建成的語義網絡,去儲存了 ... 於 medium.com -
#85.Package wordnet - CRAN
An interface to WordNet using the Jawbone Java API to WordNet. WordNet (<https://wordnet.princeton.edu/>) is a large lexical database of English ... 於 cran.r-project.org -
#86.WordNet - W3C Wiki
WordNet (r) is an online lexical reference system whose design is inspired by current psycholinguistic theories of human lexical memory. 於 www.w3.org -
#87.Wordnets in the World
Language Resource name Online Browsing Albanian AlbaNet YES Arabic Arabic WordNet (OMW) Multilingual (200+ languages) Open Multilingual Wordnet YES 於 globalwordnet.org -
#88.Extending WordNet with UFO foundational ontology
The WordNet [1], [2] is a large, machine-readable, lexical database that exposes different types of semantic relations between words regarding their meanings. 於 www.sciencedirect.com -
#89.NET Word Framework | C# and VB.NET Word API - Syncfusion
The .NET Word Framework is a high-performance and feature-rich framework that performs advanced Word document processing operations faster without Microsoft ... 於 www.syncfusion.com -
#90."wordnet" pack for SWI-Prolog
Package "wordnet". Title: Access to WordNet database. Rating: Not rated. Create the first rating! 於 www.swi-prolog.org -
#91.Ancient Greek WordNet
About. The Ancient Greek WordNet is an on-going collaboration between the Center for Hellenic Studies, the University of Exeter, and the University of Pavia ... 於 greekwordnet.chs.harvard.edu -
#92.How to use word Net with php - Stack Overflow
On the download page there's a download available with a command line tool. You could use this from php if you have exec enabled. 於 stackoverflow.com -
#93.A Complete Multilingual WordNet List by Language
WordNet is a lexical database that groups words into sets of synonyms called synsets, providing short definitions and usage examples, ... 於 www.certifiedchinesetranslation.com -
#94.Spire.Doc for .NET - E-Iceblue
Spire.Doc enables .NET(C#,VB.NET,ASP.NET) applications to read, modify and write Word documents without Microsoft Word. Support and Convert DOC, DOCX to PDF ... 於 www.e-iceblue.com -
#95.WordNet (r) 2.0 - DICT.TW Dictionary Taiwan
英漢字典、漢英字典、和英辭書、英英字典、英漢醫學字典、注音查詢、中文輸入法字典。 Chinese-English / English-Chinese / Japanese-English Dictionary, Webster, ... 於 dict.tw -
#96.Open English WordNet
Open English WordNet is derived from Princeton WordNet by the Open English WordNet Community and released under the Creative Commons Attribution (CC-BY) 4.0 ... 於 en-word.net -
#97.Sample usage for wordnet - NLTK
WordNet is just another NLTK corpus reader, and can be imported like this: >>> from nltk.corpus import wordnet. For more compact code, we recommend:. 於 www.nltk.org -
#98.WordNet - Fellbaum - - Wiley Online Library
Specifically, words in WordNet that are similar in meaning are interlinked by means of pointers that stand for a semantic relation. Formally, ... 於 onlinelibrary.wiley.com