UrDa的問題,我們搜遍了碩博士論文和台灣出版的書籍,推薦Urda, Sue寫的 The Art & Truth of Transformation for Women: The magic of shifting your mindset and opening your heart to consciously live a lif 和Urda, Sue,Fyler, Kathy的 Women Living Consciously Book II都 可以從中找到所需的評價。
另外網站Meet Our Top Wicker Park Dentist - Dr. Urda - Smile Science ...也說明:Doctor Urda brings a dedication to excellence and personal approach to her dental practice. Learn more about her story and credentials.
這兩本書分別來自 和所出版 。
逢甲大學 自動控制工程學系 洪三山所指導 曾子銓的 基於LVDT實現圓軸真圓度與凸輪擺線量測之研究 (2021),提出UrDa關鍵因素是什麼,來自於線性可變差動變壓器、LabVIEW、真圓度、擺線運動。
而第二篇論文國立陽明交通大學 智慧計算與科技研究所 謝君偉所指導 黃俊凱的 語意切割多層注意力模型與雜訊資料擴增 (2021),提出因為有 電腦視覺、語義分割、注意力模塊、融合尺度空間注意力、資料增強的重點而找出了 UrDa的解答。
最後網站Totême: 黑色Urda 背心則補充:单品信息: 黑色无袖方领背心,采用羊毛针织面料,罗纹针织饰边边缘。 供应商配色:Black. 88% 羊毛, 12% 涤纶. 进口. 202771F111020. 中国大陆:订单金额超过$350 ...
The Art & Truth of Transformation for Women: The magic of shifting your mindset and opening your heart to consciously live a lif
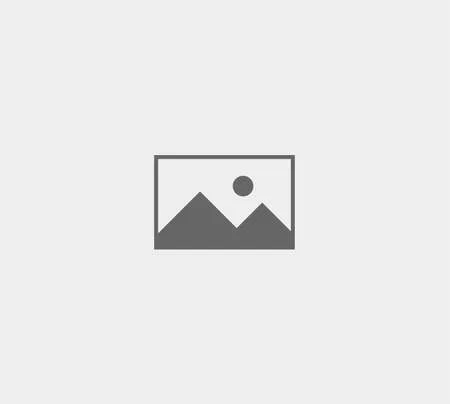
為了解決UrDa 的問題,作者Urda, Sue 這樣論述:
UrDa進入發燒排行的影片
基於LVDT實現圓軸真圓度與凸輪擺線量測之研究
為了解決UrDa 的問題,作者曾子銓 這樣論述:
從60年代起,台灣是重要的加工出口國之一,多數的外國企業都喜歡委託台灣加工廠進行產品的製作與加工;對於加工出口產品,品質的控管與檢測已成為必要審核項目。高規格的工廠在檢測上使用自動工件量測儀等量測機具進行檢測並且檢測的精度最小可以達到微米等級;但仍有多數製造工廠採用人工檢測的方式進行,以手持游標卡尺或千分表對於工件進行手動檢測。為減少人為檢測的誤差,又能在避免花費龐大金額下提升產線的效率,本研究以線性可變差動變壓器(Linear Variable Differential Transformer, LVDT)為研究主軸,證實LVDT對於工件之量測的可信度與精確度,以LVDT架構之量測系統
將比傳統之量具更加快速,且量測精度能達到跟傳統手動量具同等之精確度,在成本開銷上又比市售的量測機台來的更低。 本研究以LVDT取代傳統量具作為量測工件之主軸,選擇工件中圓軸之真圓度以及凸輪之擺線曲線作為LVDT量測目標;整合LVDT、步進馬達、鋁擠型等物件架構出測量平台,將LVDT量測到工件之徑向位移量轉變為類比電壓訊號,藉由資料擷取器將訊號送至LabVIEW人機介面中進行資料統整及運算,最終將計算出工件參數以數值或圖表形式顯示於電腦螢幕上,證實LVDT能夠達到上述之量測效果,提供一種新的量測方式。
Women Living Consciously Book II
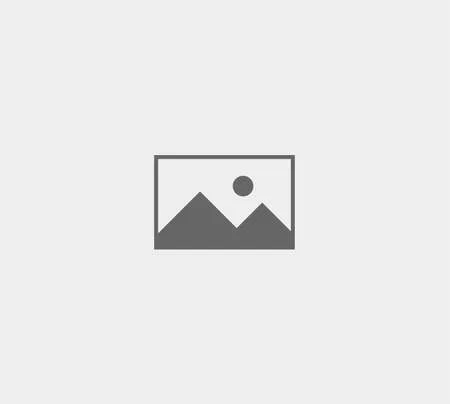
為了解決UrDa 的問題,作者Urda, Sue,Fyler, Kathy 這樣論述:
語意切割多層注意力模型與雜訊資料擴增
為了解決UrDa 的問題,作者黃俊凱 這樣論述:
目 錄摘要 iAbstract ii目 錄 iiiList of Figures viList of Tables viiiChapter 1 Introduction 1Chapter 2 Background 52.1 Semantic Segmentation 52.2 Attention Module 52.3 Data Augmentation 6Chapter 3 Methodology 73.1 SE module and SA module 73.2 Bi - Fusion Attention
Module 93.3 Bi - Fusion Attention Head on Backbone 113.4 Data Augmentation with Background Noise on the Cityscapes Dataset 123.5 Feature Expansion Layer on HRNet 133.5.1 Features are power of 2 133.5.2 Feature Expansion Layer with a Power of 1.5 143.5.3 Repeatable Feature Expansio
n Layer with a Power of 1.5 163.5.4 Feature Expansion Layer with Dilated Convolution 17Chapter 4 Experiments and Results 194.1 Dataset 194.1.1 Cityscapes dataset 194.1.2 Pascal-Context dataset 194.2 Training 194.3 BFA Results on Cityscapes Dataset 204.4 BFA Results on PASCAL-
Context dataset 224.5 Results of Data Augmentation with Background Noise 234.6 Results of Feature Expansion Layer on the HRNet 234.6.1 Feature Expansion Layer with a Power of 1.5 234.6.1 Repeatable Feature Expansion Layer with a Power of 1.5 244.6.2 Feature Expansion Layer with dilate
d convolution 244.7 All methods comparison on the Cityscapes validation set. 254.7 Ablation study on the BFA module 264.7.1 Fusion vs. Attention 264.7.2 Channel Concatenation vs. 1x1 convolution 274.7.3 Maximum Pooling vs. Average Pooling 274.7.4 Attention map addition vs. Residual
feature map addition 274.7.5 Numbers of convolution layers in BFA 284.7.6 Sigmoid function in BFA 294.8 Ablation Study on Noise weight for data augmentation 294.9 Ablation study on Feature Expansion Layer with a Power of 1.5 304.9.1 Method 1 of FEP 304.9.2 Method 2 of FEP 314.9
.3 Method 3 of FEP 324.9.4 Method 4 of FEP 334.9.5 Method 5 of FEP 344.9.6 Method 6 of FEP 354.10 Ablation study on Feature Expansion Layer with Dilated Convolution 364.10.1 Deeper features have abundant semantic information 364.10.2 Method 1 of FEDC 374.10.3 Method 2 of FEDC
384.10.4 Method 3 of FEDC 38Chapter 5 Conclusion and Future Work 39References 40
UrDa的網路口碑排行榜
-
#1.urda的中文意思 - Dict.site 英漢/漢英線上英文字典
urda 中文意思是什麼. urda 解釋. 烏爾達. 例句. 目前還沒有urda例句。 相似字; 英漢推薦; 漢英推薦. urbino etang · urc · urc uniform resource citation. 於 dict.site -
#2.請問關於學校裡Urda的使用狀況 - 中山大學板 | Dcard
請問關於學校裡Urda的使用狀況. 中山大學. 2020年7月28日04:47. 小弟台北人今年錄取中山目前沒有自己的車都騎Wemo或Goshare 上面2種是隨借隨停(只要停在合格的機車位 ... 於 www.dcard.tw -
#3.Meet Our Top Wicker Park Dentist - Dr. Urda - Smile Science ...
Doctor Urda brings a dedication to excellence and personal approach to her dental practice. Learn more about her story and credentials. 於 www.smilesciencechicago.com -
#4.Totême: 黑色Urda 背心
单品信息: 黑色无袖方领背心,采用羊毛针织面料,罗纹针织饰边边缘。 供应商配色:Black. 88% 羊毛, 12% 涤纶. 进口. 202771F111020. 中国大陆:订单金额超过$350 ... 於 www.ssense.com -
#5.Recogen firmas para que se cubran las dos plazas de médico ...
El Ayuntamiento de Urda (2.477 habitantes) ha iniciado una recogida de firmas para solicitar a la Consejería de Sanidad la cobertura ... 於 www.abc.es -
#6.Urda Eichhorst - NDC Transport Initiative for Asia
Senior Project Director, Deutsche Gesellschaft für Internationale Zusammenarbeit (GIZ) GmbH. Urda is the director of the NDC Transport ... 於 www.ndctransportinitiativeforasia.org -
#7.Artesã | Urda | Brasil
O nome Urda carrega o conceito e a tradição do tear. Propomos uma moda e uma possiblidade de decoração sustentável e colorida. 於 www.urda.com.br -
#8.Matt Urda - Booz Allen Hamilton
Matt Urda is a leader in the firm's health business specializing in information technology for clients such as the CDC. He leads the firm's Atlanta office. 於 www.boozallen.com -
#9.urda - 維基詞典,自由的多語言詞典
urda. 語言 · 監視 · 編輯. 滿語. 烏爾達. 取自「https://zh.wiktionary.org/w/index.php?title=urda&oldid=5049181」. 最後一次編輯於5 年前由OctraBot. 維基詞典. 於 zh.m.wiktionary.org -
#10.Gavin Urda - 2021 - Football - UNH Wildcats
Gavin Urda (34) Cornerback - 2021- Recorded first career tackle against Pitt (9/25/21) 於 unhwildcats.com -
#11.Harrison Urda - 2018 - Men's Lacrosse - Eastern Connecticut ...
Harrison Urda (30) Midfield - 2018: Fourth year member of the team… fourth year varsity letter winner… First team NEILA D3 All-Academic Team… 於 gowarriorathletics.com -
#12.Claudia Urda (Detail) - Kinstellar
Claudia Urda is an Associate in our Bucharest office focusing on environmental and data protection law. Her knowledge touches all areas of environmental law ... 於 www.kinstellar.com -
#13.Small paintings Mixed Urda by Vacaru Nicoleta - Carré d'artistes
Urda · Vacaru Nicoleta · Description artwork · information · Other artworks from this artist · Recently viewed products · Unique art for... · Receive our exclusive ... 於 www.carredartistes.com -
#14.Home - URDA Lebanon
URDA, Union of Relief and Development Associations, is a Lebanese non-governmental organization dedicated to ensuring the human rights of the most ... 於 urda-lb.org -
#15.Union of Relief and Development Associations - URDA
URDA, Union of Relief and Development Associations, is a Lebanese non-governmental organization dedicated to improving the human rights of the most ... 於 tw.linkedin.com -
#16.Urda最佳旅遊景點
Urda 旅遊景點:請参考Tripadvisor 上西班牙Urda景點的真實旅客評論和照片。 於 www.tripadvisor.com.tw -
#17.Jacob Urda, D.O. | Family Medicine | Crestview, Florida
Dr. Jacob Urda, D.O. at the White-Wilson Family Medicine Clinic in Crestview, Florida. 於 www.white-wilson.com -
#18.Cum se face urda de casa? (branza ricotta) | Savori Urbane
Urda sau ricotta este un subprodus lactat preparat prin re-fierberea zerului ramas de la branza (branza de vaci, de oi, cas etc). Sursa aici si ... 於 savoriurbane.com -
#19.Urda - NPC - World of Warcraft - Wowhead
Urda <Wind Rider Master> · Level: 50 - 60 · Classification: Elite · React: A H · Faction: Orgrimmar · Type: Humanoid · Added in patch 1.11.1.7272 · Health: 3,117,801 ... 於 www.wowhead.com -
#20.Best trails in Urda, Toledo | AllTrails
Explore the most popular trails near Urda with hand-curated trail maps and driving directions as well as detailed reviews and photos from hikers, ... 於 www.alltrails.com -
#21.澎湖租機車|UrDa環保電動車輕鬆遊 - KKday
1.打開Urda app · 2.搜尋附近站點,確認該站車輛的電量狀況並預約租車 · 3.輸入序號 · 4.點選啟動車輛後即可騎乘. 於 m.kkday.com -
#22.UrDa悠達趨動,雲端共享電動機車參觀 - goldentaiwan的部落格
這次跑去南港智慧城市展參觀其昜公司的UrDa電動機車共享系統。 系統的核心就是採用了其昜這台大馬力的電動機車,配合上他們的4G連網系統,使用者透過APP預約一台電量夠 ... 於 goldentaiwan.pixnet.net -
#23.urda中文 - 查查綫上辭典
urda 中文::烏爾達;于拉峰;羊陰乳清酒;于達;烏爾德…,點擊查查權威綫上辭典詳細解釋urda的中文翻譯,urda的發音,音標,用法和例句等。 於 tw.ichacha.net -
#24.ADRIANA URDA - Google Academic
Co and Ni ferrospinels as catalysts for propane total oxidation. A Urdă, A Herraïz, Á Rédey, IC Marcu. Catalysis Communications 10 (13), 1651-1655, 2009. 於 scholar.google.com -
#25.悠達共享系統型錄/UrDa Sharing System Brochure - AHAMANI ...
悠達智慧共享電動機車租賃系統中英文型錄. UrDa Smart Sharing E-Scooter System Brochure. Copyright © 2015 ‧ AHAMANI EV TECHNOLOGY CO., LTD. Powered By web3.tw ... 於 www.ahamani.com -
#26.UrdA Controls Secondary Metabolite Production and the ...
In these organisms, secondary metabolism and morphogenesis are subject to a complex genetic regulation. Here we functionally characterized urdA, ... 於 www.ncbi.nlm.nih.gov -
#27.Weather Urda - meteoblue
Today's and tonight's professional weather forecast for Urda. Precipitation radar, HD satellite images, and current weather warnings, hourly temperature, ... 於 www.meteoblue.com -
#28.URDA OD SURUTKE MLADOG SIRA - Coolinarika
Svaki put kad pravim "domaci sir od sirista"https://www.coolinarika.com/recept/domaci-sir-od-sirista/ iskoristim da napravim cuvenu gospodju ... 於 www.coolinarika.com -
#29.31 Steve Urda - Baseball Coach - Slippery Rock University ...
Former Rock pitching standout Steve Urda finished his seventh year as an assistant to and pitching coach for veteran Rock head coach Jeff Messer in 2011. 於 rockathletics.com -
#30.Urda Natures Promise 400g - Maxi
Koliko vam se dopalo? Ocenite ovaj proizvod. ID: 7534303. *Delhaize nastoji da dobije tačne i pouzdane podatke o proizvodima („Podaci“) ... 於 www.maxi.rs -
#31.Urda Eichhorst - Changing Transport
Urda Eichhorst. Project Director NDC Transport Initiative for Asia Based in Bonn, Germany. Currently, I am heading the NDC Transport Initiative for Asia, ... 於 changing-transport.org -
#32.Urda | Local Cheese From Romania - TasteAtlas
Urda is a fresh whey cheese produced throughout the Balkans. The cheese is made from the whey of cow's, sheep's, or goat's milk. Its texture is smooth, ... 於 www.tasteatlas.com -
#33.Urda - EKOFIL Mlekara Homolje
Sa Homolja nam dolazi i sir urda. Sir urda se uglavnom vezuje za domaću radinost i teško pronalaženje u marketima. 於 www.ekofil.co.rs -
#34.Urda, West Kazakhstan Region, 哈薩克目前天氣 - AccuWeather
為當天做好準備。使用雷達、每小時和最新天氣預報檢查未來一天Urda, West Kazakhstan Region, 哈薩克的目前天氣。 於 www.accuweather.com -
#35.Rastislav Urda Tennis Player Profile | ITF
Rastislav Urda (35) is a tennis player from Slovakia. Click here for a full player profile. 於 www.itftennis.com -
#36.Urda, 1 kg, Laktotrio, Produs in Bucovina, 100% natural
Urda contine cea mai putina grasime si este bogata in saruri minerale si proteine de calitate. 於 www.laktotrio.ro -
#37.Urda Project - SoundCloud
Play Urda Project on SoundCloud and discover followers on SoundCloud | Stream tracks, albums, playlists on desktop and mobile. 於 soundcloud.com -
#38.Urda dulce de Ibanesti Mirdatod 500 g - Auchan online
Cumpara Urda dulce de Ibanesti Mirdatod 500 g online de la Auchan, calitate exceptionala la pret avantajos. Ridicare rapida! Drept de retur in 14 de zile. 於 www.auchan.ro -
#39.Urda | Suikoden Wikia
Urda is the Chisou Star in Suikoden V. Like the other elves, Urda think that human is barbarous. She lives in Alseid and like most elf Urda prefer bow as ... 於 suikoden.fandom.com -
#40.Jennifer Urda / Welcome - Wilson County Schools
Wilson County Schools does not discriminate on the basis of race, color, national origin, sex, disability, marital or parental status, in admission, to access, ... 於 www.wilsonschoolsnc.net -
#41.Peter Urda urda - GitHub
Your friendly West Coast Software Engineer. urda has 4 repositories available. Follow their code on GitHub. 於 github.com -
#42.Urda by Breyten Breytenbach on Amazon Music
Check out Urda by Breyten Breytenbach on Amazon Music. Stream ad-free or purchase CD's and MP3s now on Amazon.com. 於 www.amazon.com -
#43.Urda, Richard B., Jr. - Board Certified Indiana Trust & Estate ...
Urda, Richard B., Jr. Employee Benefits, Estate Planning and Administration, Small Business Law and Professional Corporations Urda Professional Corporation ... 於 indianatrustestatelawyers.org -
#44.Descripción de la fiesta Santísimo Cristo de Urda | TCLM
Urda, el pueblo que se recoge en las faldas de los Montes de Toledo, al abrigo de La Calderina, su pico más alto, y se abre, como una metáfora del espíritu ... 於 www.turismocastillalamancha.es -
#45.Dr. Julie Urda - Rhode Island College
Julie Urda received her Ph. D. in Management concentrating in Organizational Behavior in 2006 from INSEAD, an international business school in Fontainebleau ... 於 www.ric.edu -
#46.Union of Relief and Development Associations - URDA
Union of Relief and Development Associations - URDA · Our Vision. We aspire to become the safest refuge for all the disadvantaged individuals in ... 於 daleel-madani.org -
#47.Tyler Urda, Business Development Representative - Sea Limited
Tyler Urda, Business Development Representative. Since 1970, S.E.A., Ltd. has provided multi-disciplined forensic engineering, fire investigations and ... 於 sealimited.com -
#48.Raum Urda-Stein - OSRS Wiki
Raum Urda-Stein is the owner of Armour Shop, a small armour store on the Fremennik island of Jatizso. Raum also plays a minor role in The ... 於 oldschool.runescape.wiki -
#49.Cynthia Urda Kassis | Shearman & Sterling
Cynthia Urda Kassis is a Lead Industry Coordinator for Mining & Metals and a partner in the Project Development & Finance practice. 於 www.shearman.com -
#50.Susana Ortiz-Urda, MD, PhD, MBA - UCSF Helen Diller Family ...
Susana Ortiz-Urda, MD, PhD, MBA. Associate Professor, Department of Dermatology, UCSF. Cancer Center Program Membership. Molecular Oncology. 於 cancer.ucsf.edu -
#51.Dr. Monica Urda, DDS, Chicago, IL | Dentist | Get Virtual Care
Book an appointment online now with Dr. Monica Urda, DDS of Chicago, IL (60622). Read verified patient reviews and make an appointment instantly. 於 www.zocdoc.com -
#52.作者Urda 的總覽(PTT發文,留言,暱稱) - PTT網頁版
234 F 噓Urda: 跟胃導一樣都是爵卿炒出來的過譽仔看她在那裝可愛就覺尷尬05/18 21:05. [新聞] 女英票房之冠回歸!《驚奇隊長2》5月開拍. 於 www.pttweb.cc -
#53.Urda 悠達趨動
UrDa 是透過智慧雲端裝置與電動機車的結合,創造一個電動機車共享租賃服務。可有效減少汽油機車的自購率,解決空污及噪音、交通壅塞等問題,並實踐「共享經濟」的理念。 於 urdamoto.com -
#54.三個騎UrDa 前就爛到不行的體驗: 從前端工程師的角度淺談如何 ...
三個騎UrDa 前就爛到不行的體驗. 前陣子跟了一波報復性出遊,終於到澎湖看了心心念念快十年的花火節,當年大學歡樂畢旅腦筋轉不過來想不開,竟然傻呼 ... 於 linyencheng.github.io -
#55.Kathleen E. Urda - Jane Austen Society of North America
Going from Extremes: Mansfield Park as a Revision of Clarissa. Kathleen E. Urda. Kathleen E. Urda (email: [email protected]) is Assistant Professor ... 於 jasna.org -
#56.Urda sir: pun proteina, skoro bez masti - NaDijeti.com
Urda sir je albuminski sir proizveden od surutke. Svež, grudvast, pomalo suv i bez masnoće urda je prava stvar za mnoge dijete, ... 於 nadijeti.com -
#57.在App Store 上的「UrDa」
閱讀評論、比較客戶評分、查看截圖,並進一步瞭解「UrDa」。下載「UrDa」並在iPhone、iPad 和iPod touch 上盡享豐富功能。 於 apps.apple.com -
#58.Search hotels in Urda
Apartamento Rural Los Laureles is set in Urda in the Castilla-La Mancha Region, 65 km from Toledo. Daimiel is 39 km from the property. 於 www.booking.com -
#59.【Urda 悠達趨動】信用卡最優10%|共0家銀行有刷卡優惠整理
iCard.AI推薦Urda 悠達趨動信用卡優惠前5名,安泰Living Green綠活卡最優10%,最新、限時活動、支付優惠、刷卡回饋懶人包、比較|iCard. 於 icard.ai -
#61.Beatriz Urda | BSC-CNS
Beatriz Urda. Beatriz Urda's picture. BEATRIZ URDA; Position: RESEARCH STUDENT; Barcelona Supercomputing Center - Centro Nacional de Supercomputación Life ... 於 www.bsc.es -
#62.Ultra Rapid Deployable Antenna (URDA) - esa artes
Ultra Rapid Deployable Antenna (URDA) ... When disaster has struck, applying correct aid and relief work is critical, in particular within the first few hours and ... 於 artes.esa.int -
#63.Urda - MyAnimeList.net
Looking for information on the anime Urda? Find out more with MyAnimeList, the world's most active online anime and manga community and database. 於 myanimelist.net -
#64.(Urda). - Urdă cheese on Catalog-Cs.Info
Urda is a type of traditional whey cheese whose recipe has been passed from generation to generation in most households. It is common in the Balkans region, ... 於 catalog-cs.info -
#65.UrDa悠達共享電動機車- 首頁 - Facebook
UrDa 悠達共享電動機車, 高雄市。 1255 個讚· 18 人正在談論這個。 UrDa悠達服務特色: 1、App即您的鑰匙。 2、採固定式站點:可甲地借乙地還。 於 zh-tw.facebook.com -
#66.Urda-sitan sir za detoksikaciju! - GOMEX
Urda je visokoproteinski nemasni sir. Liči na tzv. “švapski”, ali je suvlji i grudvastiji. Blažeg je ukusa, nije kiseo i pravi se ... 於 www.gomex.rs -
#67.URDA cloud sharing electric motorcycle leasing system
The URDA cloud sharing electric motorcycle leasing system is a business service model. The hardware part of the system includes electric motorcycles and ... 於 en.smartcity.org.tw -
#68.Sir urda alternativa 400 g vakum Olpa - Voli eCommerce
Nacionalni trgovački lanac Voli odlikuju vrhunska usluga, ugodna kupovina u prijatnom ambijentu i velika ponuda kvalitetnih proizvoda po povoljnim cijenama. 於 voli.me -
#69.其昜電動車科技- UrDa智慧電動車系統獲政府肯定
未上市股票撮合,0938-826-852 張先生UrDa智慧電動車系統獲政府肯定其易電動車科技股份有限公司以環保與再生能源出發點,今年以UrDa悠達雲端共享電動機車租賃系統, ... 於 kuangyi01.pixnet.net -
#70.【Urda住宿訂房推薦】最新特惠&住客真心話評鑑|Agoda.com
Urda 飯店、民宿、青年旅館、Villa、公寓...等各類住宿比價。Urda熱門住宿: 於 www.agoda.com -
#71.高雄環保共享電動機車騎乘心得(UrDa) - Mobile01
今天我要來跟大家介紹一下高雄環保的UrDa悠達共享電動機車系統!目前使用過悠達兩次,以下分別會介紹UrDa基本的使用方式以及心得(・∀・)つ⑩北有WeMo ... 於 www.mobile01.com -
#72.Urda - Weather forecast - Yr
Urda. Toledo, Castille-La Mancha (Spain), elevation 770 m. Press to show information about this location. ForecastForecast. 於 www.yr.no -
#73.Urda - Grupo de Desarrollo Rural Montes de Toledo
Urda se enclava entre las sierras de La Calderina y los Morrones, ... La Sagrada Imagen del Cristo de la Vera Cruz o Cristo de Urda es una talla de la ... 於 www.montesdetoledo.net -
#74.Urocanate reductase - urdA gene & protein - UniProt
urdA. Organism. Shewanella oneidensis (strain MR-1). Status. Reviewed-Annotation score: Annotation score:4 out of 5. The annotation score provides a ... 於 www.uniprot.org -
#75.電動機車點數使用篇-UrDa悠達趨動 - MeN Go
UrDa 悠達趨動以低碳校園建構為基礎,延伸發展為綠能•智慧•低碳城市,規畫校園電動機車共享租賃應用服務先導運行,透過示範運行了解校園租用車輛需求與行為分析,進而建構 ... 於 www.men-go.tw -
#76.urda - Translation into English - examples Romanian
Translations in context of "urda" in Romanian-English from Reverso Context: Paine prăjita, urda, cafea cu lapte. 於 context.reverso.net -
#77.Urda Name Meaning & Urda Family History at Ancestry.com®
Discover the meaning of the Urda name on Ancestry®. Find your family's origin in the United States, average life expectancy, most common occupation, ... 於 www.ancestry.com -
#78.Susana Ortiz-Urda | UCSF Health
Susana Ortiz-Urda, MD, is a dermatologist and melanoma specialist practicing at UCSF Health. Learn more about Dr. Ortiz-Urda. 於 www.ucsfhealth.org -
#79.UrDa悠達智慧雲端共享電動機車租賃系統- Google Play 應用程式
您好~ 歡迎加入UrDa悠達智慧雲端共享電動機車租賃系統您可透過本App完成線上預約及租借等服務※目前僅開放國立中山大學校區進行試營運建議您可瀏覽我們的官方網站或直接 ... 於 play.google.com -
#80.UrDa悠達智慧共享電動車 - LINE熱點
UrDa 是透過智慧雲端裝置與電動機車的結合,創造一個電動機車共享租賃服務。可有效減少汽油機車的自購率,解決空污及噪音和交通壅塞等問題,並實踐「共享經濟」的理念. 於 spot.line.me -
#81.URDA (Pleasure Craft) Registered in Norway - Marine Traffic
Where is the ship? Pleasure Craft URDA is currently located at NORDIC - Skagerrak at position 59° 54' 39.276" N, 10° 42' 3.456" E as reported by MarineTraffic ... 於 www.marinetraffic.com -
#83.Urda: cum se prepara si ce beneficii are pentru organism
Urda este formată în bună parte din lactalbumina laptelui, care nu precipită sub acţiunea cheagului şi trece în zer. Prin încălzirea zerului la ... 於 cultivaprofitabil.ro -
#84.URDA:The Third Reich - ak8062的創作- 巴哈姆特
URDA :The Third Reich. 作者:クロガネ│2011-06-23 16:22:45│巴幣:0│人氣:195. 昨天半夜都要睡了的時候偶然在英文維基發現了一部WEB動畫 大約是2002~2003的作品 於 home.gamer.com.tw -
#85.Urda E-scooter - Instagram
19 Followers, 8 Following, 69 Posts - See Instagram photos and videos from Urda E-scooter (@urda_escooter) 於 www.instagram.com -
#86.Urda (Toledo) - 7-Day weather forecast - Table - AEMET
Weather. Forecast for Spanish towns: Urda (Toledo) - 7-Day weather forecast - Table. 於 www.aemet.es -
#87.Urda (journal) - Wikipedia
Urda was a Norwegian antiquities and history journal published from 1834 to 1849 by the University Museum of Bergen, which wanted to convey what the museum ... 於 en.wikipedia.org -
#88.Sour Urda - Arca del Gusto - Slow Food Foundation
Urda or whey cheese belongs to category of fresh cheeses produced from raw sheep's milk. It has soft, spreadable consistency with a characteristic sour. 於 www.fondazioneslowfood.com -
#89.Urda Dal 500gm - MyyShopp.com
Buy Urda Dal 500gm - Loose Item - 500 gm from Welcome Super Market at Rs.0.00 Shop online in Mumbai Maharashtra Urad Dal and get home delivery at lowest ... 於 www.myyshopp.com -
#90.Urda Images, Stock Photos & Vectors | Shutterstock
Find urda stock images in HD and millions of other royalty-free stock photos, illustrations and vectors in the Shutterstock collection. 於 www.shutterstock.com -
#91.Property for sale in Urda, Toledo, Spain: 38 houses and flats
38 houses and flats for sale in Urda, Spain, from 18000 euros. Property in Urda for sale direct from owners and real estate agents. idealista, the leading ... 於 www.idealista.com -
#92.Urda - Produse piata - alimentatie - OLX.ro
urda in categoria Lactate; urda in categoria Agro si industrie; urda in categoria Alte produse alimentare. Doresti sa salvezi aceste criterii de cautare? 於 www.olx.ro -
#93.Partner - URDA Lebanon - UNHCR data portal
URDA Lebanon. URDA, Union of Relief and Development Associations, is a Lebanese non-governmental organization dedicated to ensuring the human rights of the ... 於 data2.unhcr.org -
#94.【UrDa悠達趨動】綠能智慧悠遊高雄套票(KHH13)
【UrDa悠達趨動】綠能智慧悠遊高雄套票(KHH13). done_all. 共享智慧生活,低碳環保新旅行. done_all. 交通全包,自駕暢遊. done_all. 電動機車自駕,暢遊景點不受限. 於 kw-travel.com.tw